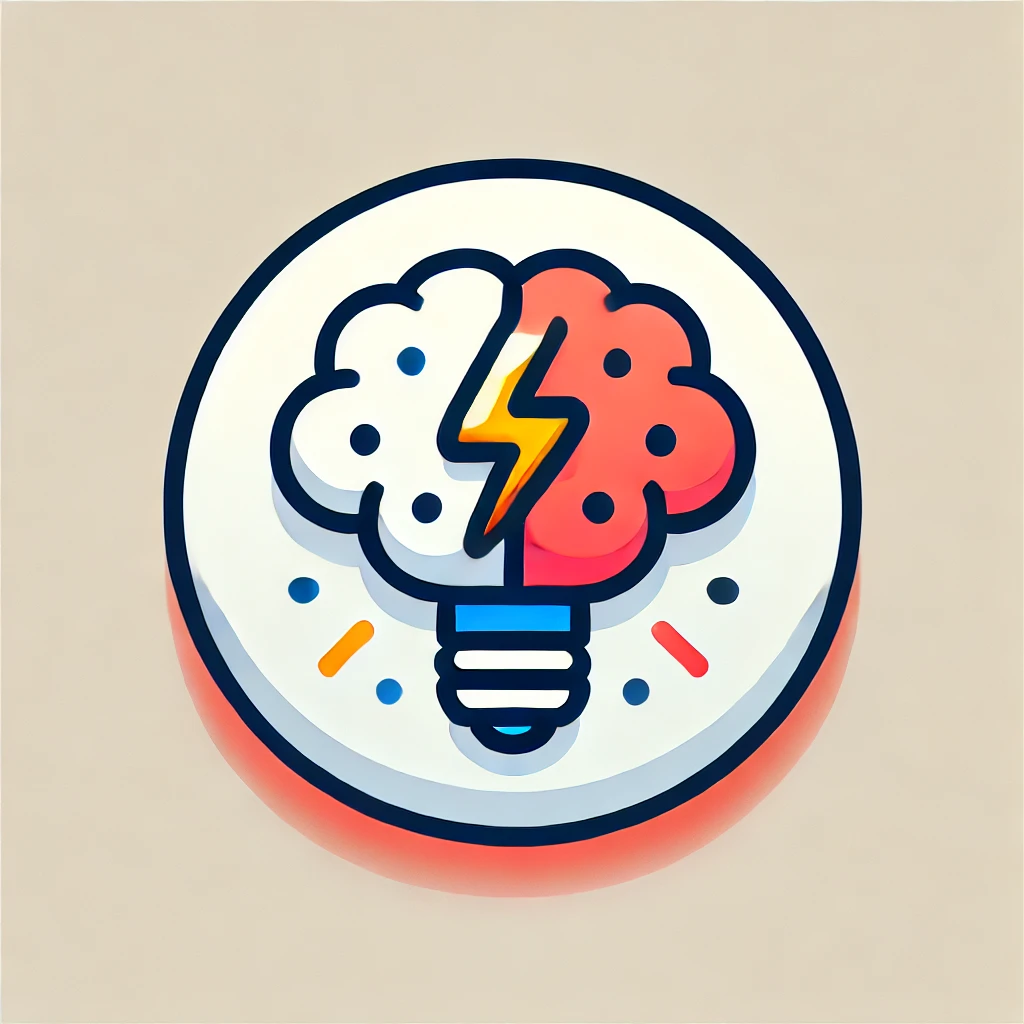
Adversarial Robustness
Adversarial robustness refers to how well a machine learning system, like a facial recognition or spam filter, can withstand deliberate attempts to deceive it. Sometimes, small, carefully crafted changes—imperceptible to humans—can trick the system into making wrong decisions. Building adversarial robustness means designing the system so it remains reliable and accurate even when facing such tricky inputs. It's like strengthening a lock so that it can't be easily bypassed by someone trying to pick it with clever tricks, ensuring the system's performance stays stable against malicious attempts.